A survey of North American insurance professionals reveals widespread use of predictive analytics in the property/casualty insurance industry, with as many as 82 percent responding that they use predictive modeling in one or more lines.
Earnix, a provider of integrated pricing and customer analytics solutions for banking and insurance, and ISO, a source of information about property/casualty insurance risk and a member of Verisk Insurance Solutions group at Verisk Analytics, earlier this month released the results of a joint industry survey, 2013 Insurance Predictive Modeling Survey. The firms compiled responses collected online in September from 269 insurance professionals representing companies that sell personal and commercial coverage in Canada and the United States.
While only 18 percent of the professionals surveyed said their companies did not use predictive analytics in any line of business, the most common line for the remainder was personal auto (49 percent). Lines like commercial property and even workers compensation and general liability were also indicated by some survey respondents.
Professionals surveyed are mainly involved in actuarial, underwriting or product development functions.
Based on the survey results, Earnix and ISO report that larger insurance companies are more likely to make use of predictive modeling than smaller ones. In fact, 100 percent of the respondents from companies that write more than $1 billion of gross premiums in personal insurance use predictive modeling, compared with 69 percent of the smaller personal lines writers.
The gap is narrower for commercial lines, where 75 percent of respondents for smaller companies (those writing less than $1 billion) and 88 percent of respondents from larger companies (those writing more than $1 billion) said they use predictive models.
The most common uses of predictive modeling are in pricing (loss cost modeling and tiering) and underwriting, while analytics are used to a lesser extent for marketing, claims (fraud investigation, claims forecasting and triaging) and reserving.
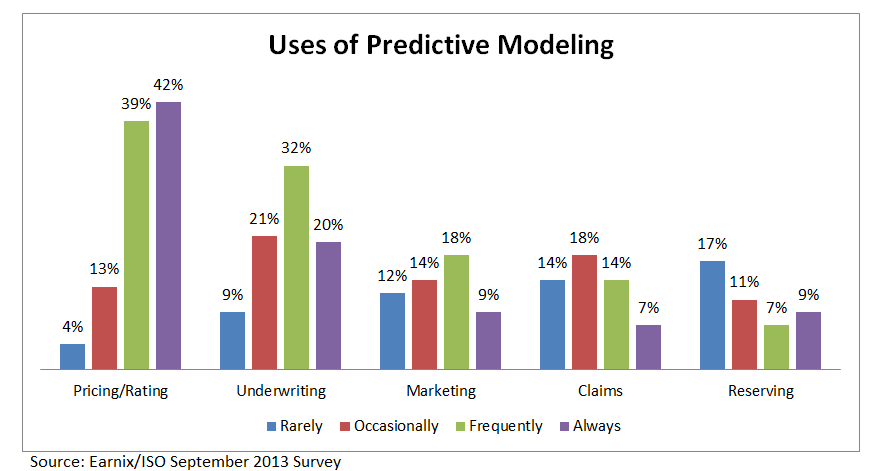
Benefits cited by survey respondents are:
- Driving profitability, 85 percent
- Reducing risk, 55 percent
- Growing revenue, 52 percent
- Improving operational efficiency, 39 percent.
Top challenges mentioned by respondents include lack of sufficient data and limited numbers of skilled modelers.
The survey also revealed that companies spend considerable time on data preparation and deployment before and after actual modeling work. More than half of survey respondents (54 percent) spend more than three months on data extraction and preparation, and more than two-thirds of the respondents (69 percent) take more than three months to deploy new models.
The complete survey report also includes responses to questions about whether external or internal resources are used to develop models (broken down by company size), primary functions responsible for modeling, the number of people devoted to the effort (by size of company), the extent to which external data are used (and type of external data used), and popular data extraction and software tools.
Source: Earnix and ISO